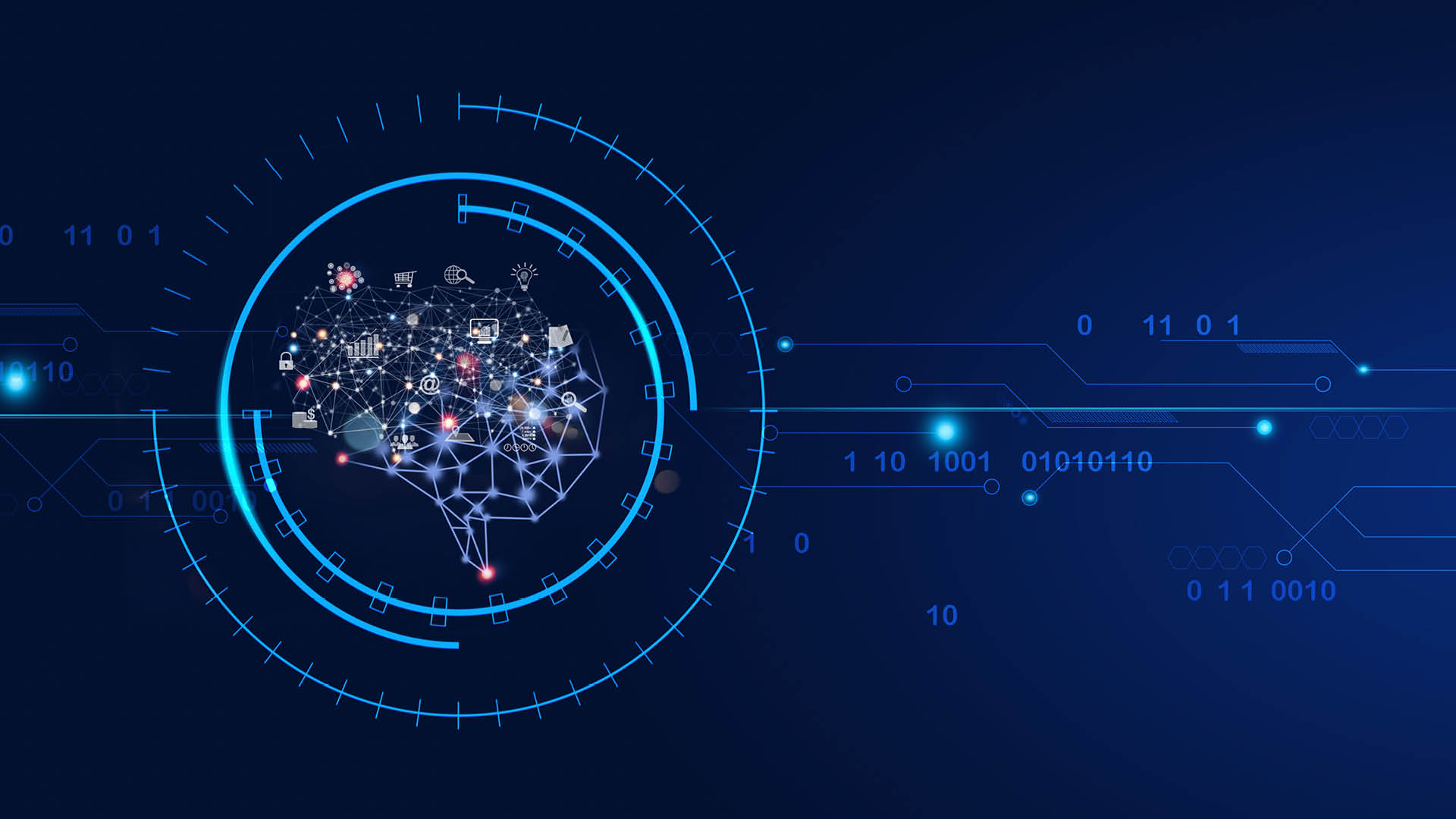
Machine learning (ML) is becoming one of the most vital assets for data security. Threat actors innovate daily, compromising data stores with greater frequency and severity than ever. ML learns trends and adapts to fight dangers to data with automation and precision. Here are some applications that explore ML’s value to data security so you can implement it appropriately to gain the full benefits.
Detecting Anomalies and Intrusions
Manual data security analysis is time-consuming and emotionally debilitating to analysts. Alert fatigue wears on them as too many false positives generate complacency. ML algorithms can be more attuned to anomalies and intrusions than human analysts.
The average data breach cost in the U.S. rose to $4.45 million in 2023, indicating data attack severity and frequency. Perpetual data security job vacancies compound the need for assistance.
Organizations leveraging AI and ML alongside data security teams can save $1.76 million annually. It is possible because ML algorithms learn threat trends to supplement manual detection and incident response. ML is more accurate because big data processing gives it an understanding of historical data alongside current insights. The more training and supervising data and reinforcement scientists provide, the better it can assist risk management, identification and remediation.
Predictive threat intelligence like this boosts the efficacy of data loss prevention tactics. ML could offer the same attention to detail in identifying novel disruptions because of traffic deviations or atypical user behavior. Employing ML is essential for alleviating burdens on data security analysts.
Behavioral Biometrics for User Authentication
ML learns more than external threat behaviors — it also identifies internal trends to make its awareness more comprehensive. In doing so, ML algorithms can create user biometric profiles for authentication. It can know when employees log in and what data they typically access, especially if it is high-risk.
A research study explored ML’s accuracy in using biometric profiles to identify behaviors during online card payment processing. It learned mouse and keystroke patterns from users. Specific program testing resulted in 100% accuracy in authenticating users. ML adapts and updates biometric profiles over time to ensure fewer false positives. For example, if an employee’s schedule changes to the night shift, the algorithm will realize this and authenticate the user to verify this behavior is nonthreatening.
Informed Risk Management Prioritization
Crafting a holistic, curated data security plan takes time. In the formulation stages, you must boost defenses for the data threats you are most susceptible to. ML can note each network entry request. If most malicious entries have specific signatures or behaviors, people in IT and data security can formulate strategies against the most threateninginfluences.
You may see ML noticing a high rate of botnet attacks and ransomware. Then, data analysts can focus on specific guards for these problems instead of spreading resources too thinly. Strategizing this way addresses the most prominent percentage of top threats early on, reducing pressures to protect against everything immediately.
Using ML for prioritizing can increase workforce morale. It provides specific directives with meaningful results in a landscape where triumphs are often difficult to measure. Dealing with the most high-profile dangers and seeing their rates fall based on ML analytics empowers teams to keep fighting against future threats.
Privacy-Preserving Data Analysis
Besides automated detection and understanding of users, ML is arguably the most powerful in its analytic abilities. Data analysis will assist with data visibility, threat detection, pattern identification efficacy and compliance adherence. It can stay updated on privacy regulations and send notifications to analysts when it is time to update.
The power of these numbers is crucial for keeping tabs on privacy in real time, mainly in coworking environments. ML helps with additional techniques for local privacy enhancement, such as federated learning (FL). FL tends to noncentralized data but keeps things it learns on central servers.
ML can keep privacy at the forefront if data analysts use differential privacy. ML could pore through countless data points and share that information with an entire company without revealing private information. Removing personally identifiable information is essential for massive surveys like the Census that collate vast data sets but need to share information without revealing specifics.
These tactics are only a few ways to increase internal security and privacy while reducing what threat actors might be able to uncover. Additionally, ML eliminates opportunities for human error, such as unintentionally publishing sensitive information. ML privacy efforts reduce mistakes and breaches.
Preemptive Application-Level Security
Despite all of the ways that ML and AI can help to further improve data security, in the end they are only as good as the data that they are consuming. If data is manipulated through an attack called “adversarial noise,” AI and ML could potentially not be able to properly analyze the data. However, through the use of an application-level security like MTE Technology, data is protected in the application layer, before it gets to the edge, preserving the integrity of the data.
MTE technology is a data security solution that provides application-level security through instantly obsolete, meaningless, random streams of values that can be used to replace any form of data transmitted between endpoints. This level of added security helps to maximize the potential of data security with ML and AI.
When Machine Learning Becomes Smarter Than Hackers
Cybercriminals will not extract, encrypt or delete your data if ML algorithms know the best ways to keep information safe. ML’s variety is its strength, and engineers must continue researching its abilities to unlock more data security capabilities. Detecting anomalies, identifying user behavior, automating processes and preserving privacy are essential for helping data analysts stay accurate and motivated during threats.
Written by: Zachary Amos, Contributor